Legal Geek London: HighQ Revolutionizes Contract Review and Analysis
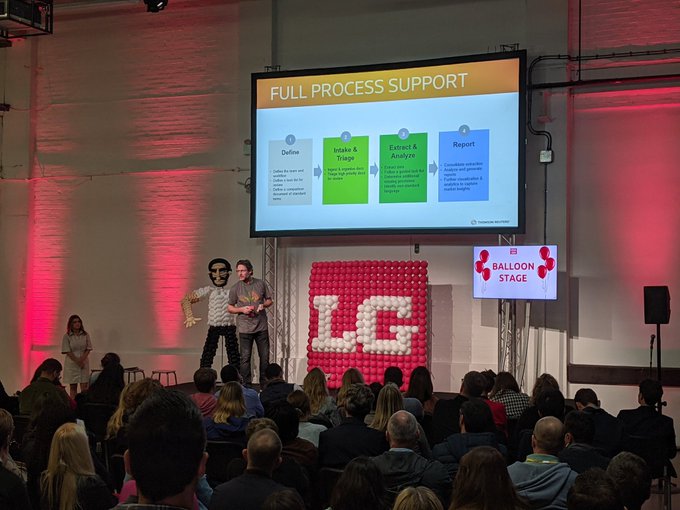
Thought leadership is the focus on Day One of the Legal Geek Conference, and a highlight of today’s sessions included HighQ Contract Analysis: The next revolution in contract review and analysis. Hosted by Steve Fullerton, senior product manager, Thomson Reuters, the session explored how HighQ Contract Analysis helps legal professionals with the contract review and analysis process.
Thomson Reuters announced new integrations and enhancements to HighQ today, including updates to the contract analysis review screen. Legal Current caught up with Fullerton after his session, and below is a recap of the conversation.
Legal Current: Why is it important to explore new approaches to contract review and analysis?
Fullerton: “Contracts and related legal documents are at the heart of most transactional workflows. Understandably, lawyers spend a lot of time reading, reviewing and creating contracts. And they spend a considerable amount of billable hours doing so. When you look at the volume of documents involved, it provides an idea of the range of use cases for contract analysis capabilities and explains why there has been considerable investment in them.
The good news is that there are a number of tools already in existence being used actively by law firms and their clients, which enable the triage of contracts and understanding of the key clauses and risks within them. They may use different machine learning methods, and some have professional services elements. But they don’t hit all the sweet spots when it comes to identifying key legal terminology throughout a document or comparing extracted language to standard terms for risk analysis.”
LC: Describe how HighQ approaches contract review and analysis.
Fullerton: “First, we are training our machine-learning algorithms to answer legal questions, not to simply identify clauses or extract entities, dates and other facts. Our models are trained upon and look to extract discrete snippets of legal language within clauses to get to the point of answering these questions – using ‘intelligent search’ algorithms created by our data scientists and training data supplied by our Practical Law subject matter experts. Moreover, wherever we find the legal language we are looking for, throughout the document, we extract and show it. This helps the reviewer to better understand multiple references to obligations, which is important where meaning or interpretation changes or is superseded.”
LC: How is HighQ using AI to help users assess contractual terms and associated risk?
Fullerton: “A big challenge for AI is the complexity and the variety of the legal language used to express the same meaning in a clause, provision or even a single sentence – or two versions where the meanings are so close to the same thing that the lawyer believes they are effectively the same; any AI will need to ignore those minor differences. An additional problem is that two lawyers may not necessarily agree on the meaning and significance of a single clause or provision.
Thomson Reuters goes a step further than extracting legal language to compare those extractions to standard terms, pre-loaded in a Practical Law standard document. We also allow customers to upload and apply their own for use and re-use across projects. The result of our comparison matching is that language-matched snippets can be viewed side-by-side within their documents, showing them in context, allowing reviewers to check for significant or risky deviations from the standard terms, including changes in obligations, and so on, to aid their overall risk assessment and reporting.”
LC: How is HighQ saving users time and effort beyond assessing the AI-extracted data?
Fullerton: “A brand new user experience in HighQ is the Contract Analysis guided review page, which shows a task-list of logically grouped questions and extracted definitions to focus the review process. It’s the feature we have focused on for much of our user experience development. It allows users to see the extracted information gathered by the machine-learning algorithms in the context of the document itself, with simple navigation from question to question, the ability to comment, amend, and validate, flag risks, plus the ability to view and download a report of the important questions you wish to output.
Watch Legal Current and follow @LegalCurrent on social for updates during the Legal Geek Conference.