Generative AI Insights from Joel Hron: How Thomson Reuters Will Deliver Trustworthy Results

Joel Hron, vice president of Technology, was among the presenters at the webinar Thomson Reuters hosted in June to offer sneak peeks of the generative AI capabilities in Westlaw, Practical Law, Legal Drafting tools, and the plugin with Microsoft 365 Copilot. More than 150 attendees submitted questions during the webinar.
Today, Hron provides the second piece in a multi-part series of Thomson Reuters subject matter experts answering attendees’ questions. He shares how Thomson Reuters is ensuring that its generative AI solutions will deliver trustworthy and accurate results.
What guardrails is Thomson Reuters putting in place to prevent hallucinations? With regards to RAG, how effective is this technique in reducing hallucinations?
Hron: “Thomson Reuters primarily uses a technique called Retrieval Augmented Generation (RAG) to help reduce errors by grounding large language models (LLMs) with reliable content. We employ this technique in a variety of pre-processing and post-processing activities to provide accurate responses. This technique highlights a key advantage for Thomson Reuters: its decades of experience and expertise in acquiring, enhancing, and managing content, and its proprietary search algorithms for retrieving the best content for the use case at hand. LLMs are trained to generate plausible text; they do this by predicting words in sequence. But this sequential prediction is purely based on probabilities. The models themselves have no grounding in anything truthful or factual and hence are prone to hallucinate – i.e., make up information. To ground these models in truth, you need to inject truth into the model through the prompt itself. For example, when a user asks a question of Ask Westlaw, our technology first runs a Westlaw search, or a series of searches, and these results are ranked based on relevance to the question being asked. The LLM then is constrained to answer the question based only on those top search results selected by our search algorithms.”
How does Thomson Reuters source the data that their LLMs are trained on?
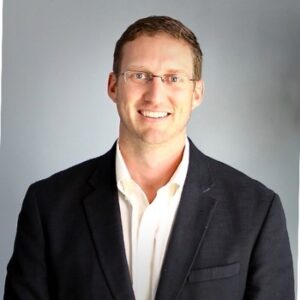
Joel Hron, Vice President, Technology
Hron: “Our approach to generative AI starts with our customers. And we understand both the risks and concerns many have in ensuring the security and privacy of their information. The Thomson Reuters advantage is that we collect and generate a significant amount of proprietary information independently, which we use to enhance and improve our LLM technologies. Our proprietary content databases and our human expertise for training and tuning AI models are critical to our training efforts. Furthermore, we are committed to unlocking the potential of AI in a transparent, ethical, and responsible way. Even before the advent of generative AI, we have developed and maintained a rigorous set of data and AI ethics principles to promote and ensure trustworthiness in its design, development, and deployment, delivering the standards for accuracy and security customers have come to expect from Thomson Reuters. This is reflected in our recently updated Data and AI Ethics Principles.”
How can you at least assure that if we use AI we are not getting false, incomplete or other erroneous results?
Hron: “Thomson Reuters believes that the role of AI is to augment and enhance the human action, not replace it. AI will never be 100% accurate. But in order to understand where and how humans should work with AI, Thomson Reuters is committed to delivering transparency and explainability into its models. For instance, many of our generative AI solutions will include inline citations to direct sources of trusted content and information used by the LLM to generate its answers. In this way, users will be able to critically validate and evaluate answers from the AI to ensure they are accurate and relevant.”
If AI is being trained on falsehoods, and is then being used to create more material from which other AI variants are trained, how can we stem the tide of falsehoods?
Hron: “Ethical implications go back to the model itself. Models are trained to generate plausible sounding text. Any question you ask, the answer will sound good. This is the beauty of these models, in fact, that they are able to generate such eloquent and grammatical responses. The problem is that these responses are not grounded in truth. To control for that, we have to build systems like RAG that ground responses in truthful and trusted sources of information. We also have to constrain the model to respond to topics for which it has truthful information and to refuse answering when it does not have the appropriate information needed to generate a truthful response. We need to constrain dialogues to the topic areas they are intended to work for – staying within the right lane.”
The elephant in the room … Will Thomson Reuters use of ChatGPT produce fake case cites as it did in the nationally publicized SDNY case of Mata v. Avianca?
Hron: “No. Using the RAG technique helps us reduce errors by grounding LLMs with reliable content. And we’re confident in our authoritative proprietary content and the technology we use for core search and verification of generative AI. Thomson Reuters has more than 150 years of cultivating legal content and more than three decades of experience in deploying AI and language models in industry-leading solutions including Westlaw and Practical Law. Our subject matter experts include 650 attorney editors supporting Practical Law and more than 1,000 attorney editors supporting Westlaw as well as thousands of experts in data science and AI software engineering.”
If AI is “reading” your draft contract, what about attorney-client privilege?
Hron: “Concerns about maintaining and preserving attorney-client privilege are, of course, top of mind for legal professionals. As we have always done, Thomson Reuters places the utmost importance on the security and privacy of our customers’ information. And we will never use customer content in a way that compromises the ethics, privacy, or best interest of our customers.”
How are you approaching security when incorporating ChatGPT into Thomson Reuters products?
Hron: “Placing trust in Thomson Reuters to manage your confidential and proprietary information is no less important than it has ever been. And Thomson Reuters has maintained a long-standing position as a leader in ensuring best-in-class security through adherence to organizational processes and controls validated through SOC 2 Type 2 auditing; benchmarking against industry-recognized standards like National Institute of Standards and Technology (NIST); and critical technical hardening through activities such as penetration testing, code and infrastructure scanning, and encryption of data at rest and in transit.”
For more insights from Hron and other Thomson Reuters leaders on shaping the future of work in the legal profession with generative AI, register here to access the recording of the June webinar. Also, sign up on TR.com/AI for updates on how Thomson Reuters is integrating generative AI across its legal solutions.